Why?
It’s no secret that media in various forms is everywhere. Thoughts, ideas, and positions bombard us from every angle and represent an ever-present demand for our attention, and increasingly our affirmation. Regrettably, these thoughts are often presented “in the heat of the moment” with the focus of embracing and espousing an emotional response; more than a carefully contemplated viewpoint. To the loss of all involved, we can also see that society in general has become less about honest dialog and discussion, with a turn to a dehumanizing or vilification of those that we disagree with.
These sources of knowledge are often quite valuable, but there is a formidable challenge in sorting through the surrounding noise and finding sources that offer the value that you are seeking. If you are seeking a “party-line” view on subjects, or, if you are looking for a genuinely felt, heated response to the latest item hot-off-the press; then please pass my blog by as noise to be filtered out. However, if you are interested in a prospective (mostly) around technical, IT-related subjects; heavily biased with considerations of the long-term impacts, considerations, and implications then I encourage you to take a look. Regardless if my monologue is about business strategy and decisions, cybersecurity, or, completely out of the technical arena to the broader questions of life, meaning or relationships; hopefully you can glean some value from my own squawking.
How?
Many of the items that I’ll be sharing may have been gleaned from environments, and sometimes second-hand knowledge where I cannot share specific details. You cannot, nor should you assume that they are all reflective of my employers, past or present. However, the lessons are (hopefully) worth repeating, so I’ll be sharing those points in a different (and often greatly simplified) context. The implementation details will obviously vary in this type of scenario, however, I will do my best to ensure that the core lessons remain unaltered.
Everyone has a set of beliefs, ideas or even biases that color how they see every subject and discussion. What I will be sharing is formed from an attempt at a holistic worldview that helps interpret what I am observing and the informing me of the implications – using the same standard in every area of life and remaining true across time. After reading my content for a short time it should come as no surprise to you that this worldview from a Christian prospective (discussed in-depth here) impacts every area of life. Specifically this is viewpoint that is based on a third-party prospective, attempting (often feebly) to be based on the authority of the Holy Scriptures in every area of life.
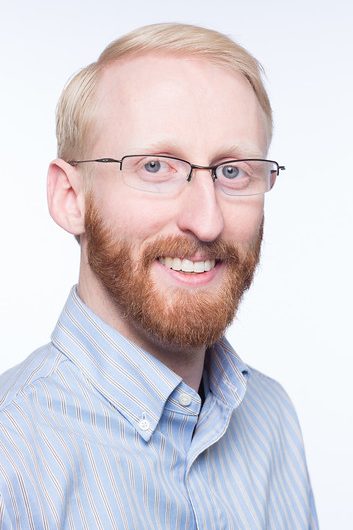
Who?
My name is Caleb and I reside in Montana with my wife and 5 children. My current occupation is serving as a Sr. Service Maturity Architect in the cloud computing space. In this role I have a bit of an odd job description, but one that I greatly appreciate. While a jack-of-all-trades and master-at-none, I do prefer the cybersecurity and audit specializations. My real-world experience is supported by currently or previously held industry certifications:
- ISACA Cybersecurity Audit Certificate
- Microsoft Certified Systems Engineer (MCSE 2003)
- AWS Solutions Architect – Associate
- Red Hat Certified Systems Administrator (RHEL 7)
- ITIL Foundations
- VMware Certified Professional
I have a few side hobbies that include hosting my own services (NextCloud, InfluxDB, Vault, HAproxy, DNS and Samba-based Active Directory, as well as things like my security-appliance-in-a-playbook) to keep technical skills alive in a context outside of my employment. Of course, they have to be properly done: built via automation (usually Ansible), by source-controlled configuration and in a manner that is worthy of cloud scale. Needless to say, details related to these environments will take up a significant portion of my posts.
My family and I also are starting to cultivate our 2.5 acre hobby farm, so there is also a bit of dabbling in Raspberry Pi-based IoT efforts to spice that up a bit.
Still interested?